Since the game has been green lit, we’ve been working mostly on the steam integration. In version 0.5, we have full support for steam cloud sync and achievements. We also incorporated user feedback concerning some visual and UI-aspects and got rid of some annoying bugs. The next version will feature some improvements to the input handling, some additional abilities for the enemies to increase diversity of behavior (even more) and whatever we get as feedback from our testers. If you want to be part of the beta program, get in touch!
Category Archives: Development
a nifty green light
When we started work on our nifty little game, there was this brand new thing Valve was trying out which would enable indie developers to get their game on Steam if the community wanted it. Steam Greenlight they called it an we thought “that’s an interesting idea, once our game is mature enough, we should try that”. Today, many years later, the day has finally come. We are happy to announce our very first, very own greenlight campaign which you can find on greenlight.aniftygame.com or in the links in the top bar. We would greatly appreciate it if you could vote for us and maybe leave a comment to tell us what you think. Thank you for your support.
Also, we are currently working on a new playtest release which should be online a few weeks from now so stay tuned…
making a procedurally generated game, what we learned (so far)
When we started the development of our nifty little game, procedural generation was slowly starting to become popular in the context of games. Now, way too many years later, procedural generation is a regularly used technique to generate content (most commonly levels) for all sorts of games. Still, few games we’ve seen have employed this technique for as many aspects of a game as we are trying to do. In this post, we want to share some of the things we learned along the way and look forward to what’s left to do to finally complete this project.
The levels
Levels are, as already discussed, the most commonly found generated aspect of games. This does not mean however, that generating (playable) levels automatically is easy to do. We went through quite some iterations of level generation techniques until we found one which worked for us. Here a brief list of the things we did in the past:
- Random room placement:
The first iteration of the level generator would randomly place rooms in an area of sufficient size. Some of the rooms were intended for special purposes, such as spawn-point, level-exit, item-room, etc., which would be rather small while other rooms were destined to contain lots of enemies and therefore had to be large. The only check it would perform at this point was that there was no overlap between the rooms. Every room had a pre-defined number of doors (depending on the pre-determined function of the room) which would lead to other rooms. After the rooms were placed, the generator would connect the rooms, ensuring that all rooms were connected to each other and that corridors would not overlap. This was done using a variant of the A*-algorithm with a special heuristic. While this worked reasonably well and offered some flexibility for parameterization of the room properties, it resulted in long corridors connecting the rooms. This might be interesting for certain types of games but since all the action in this game would happen inside the rooms, corridors were boring so having many long ones was not a good thing. We tried various things to reduce the overall corridor length and while successful to a certain degree we never were happy with the results inherently limited by the approach. - Constraint satisfaction:
Constraint Satisfaction Problems are a type of problem commonly found in the area of artificial intelligence (not the type of AI found in games though), in operations research and logistics. The idea is that you would list all the constraints which a solution to your problem would need to fulfill and let the solver come up with a solution. In our case, constraints would be things like- ‘there needs to be X rooms in this level’
- ‘every room needs to be reachable form every other room’
- ‘rooms can not overlap’
- ‘a room needs to be at least A units wide and B units high’
- ‘there must be exactly one spawn point’
- ‘there must be exactly one end point’
- ‘start-room and end-room can not be neighbors’
- ‘some rooms need to have more than two entries’ (on order to avoid that the level ends up being a chain of rooms)
- ‘the end room needs exactly one entry’
- …
The list goes on and on. The beauty of this approach is that it is extremely flexible. Once you managed to write down all your rules you can just lean back and let the machine do the rest. There is only one problem: CSP’s are hard, NP-hard. Even if you can guarantee that there is a solution to your problem, there is not necessarily an easy way to find one. While there are (somewhat) efficient ways to overcome these issues, early experiments showed that it is not feasible to generate levels this way (at least not while the player is waiting for the generation to complete) and while this approach might be interesting for, let’s say a puzzle game where you could generate the levels this way (to ensure their solvability) in an off-line fashion and distribute them to the players later, it is completely unusable for the type of game we are trying to make.
- Room Job assignment:
What we ended up with in thefinalcurrent version of the game is a bit of a compromise between the two previous approaches. The main difference between this and the previous methods is that not all constraints are considered at the beginning but processed at a later stage in a best-effort kind of way. (The technical term for this is ‘constraint relaxation’.) We would fist generate rooms and place them in a roughly grid-like pattern. Each room has a maximum on how much space it is allowed to take up, when a new room is placed next to an existing one it will take try and take up as much space as it can without surpassing this maximum or intersecting with other rooms. Once all rooms are placed this way, they get assigned jobs. Instead of defining hard constraints, each ‘Room-Job’ has a metric by which it evaluates all the as of yet unassigned rooms and picks the best (or at least the least bad) room for the job. Room jobs are prioritized such that start- and end rooms are chosen first in order to avoid constraint violation.
While this method is not guaranteed to produce the best possible levels, it always produces levels which are playable. It also has the advantage that it is fast. In fact. it is even faster than the first method described here while consistently producing superior results.
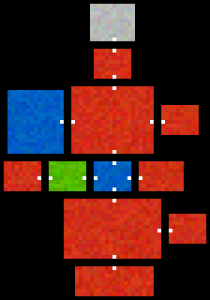
Layout of a level: start and end rooms are marked in blue, item rooms in green and trap rooms in grey.
These were not all the things we tried over the years but they were the most relevant. Now that we have a basic level, we need to fill it with stuff.
The enemies: looks
Early versions of the game had hand crafted enemies. While we were trying to figure out in what direction we wanted to go with the game, it became clear that this would not do. So we had to come up with a way to generate enemy sprites which were consistent with the overall ascetic of the game, sufficiently different from each other and still immediately recognizable as enemies. This turned out to be easier than expected. We wrote a blog post about the method a while back which goes into more detail but here is the gist of it:
- Choose a base color
- Set a seed point in the middle of the sprite
- While not enough pixels set do:
- Randomly select a pixel that’s next to a set pixel
- Compute probability for setting this pixel
- Set the pixel using the probability from 2.B
- Set a second pixel in order to assure symmetry
- Choose a highlight color given the base color
- While not enough highlights are set do:
- Select a non highlighted (base colored) pixel by random
- Highlight the pixel
- Highlight a second pixel in order to assure symmetry
- Generate 3d model from sprite
The results look something like this:
The enemies: behavior
Having enemies look different from one another is one thing, having them behave differently is an entirely different problem. We wanted the enemies not only to start out with a different behavior but also have their behavior change over time and ideally adapt it to the actions of the player. In order to accomplish this, we turned the entire game into an evolutionary optimization problem.
Each enemy has a species which has certain characteristics. These characteristics are encoded in a data structure we call the genome, loosely analogous to what happens in biology. The reason we have the ‘genome’ on species level rather than on the level of an individual is that enemies of the same species share all their properties with the only exception of their highlighting pattern as described above. Each genome has genes (obviously) which are grouped into segments. Each segment controls a different aspect of the overall behavior of the species. These segments contain (among others):
- Type: if the enemy is one entity or a swarm
- Color: the color palette containing base and highlight colors
- Health
- Size
- Level of detail: how many voxels are used in the model of the enemy given its size
- Speed
- Movement: what strategy it employs to get around
- Target selection: how it selects when and what to attack
- Aiming: the strategy describing where to aim and when to fire
- Weapon
- Shot mod: what modifiers to add to a shot
- Drop: what to drop when killed
To explain how the genes are implemented it is easiest to have a look at the code:
1 2 3 4 5 6 7 8 9 10 11 12 13 14 15 16 17 18 19 20 21 22 23 24 25 26 27 28 29 30 31 32 33 34 35 36 37 38 39 40 41 42 43 44 45 46 | public abstract class Gene { protected final Genome genome; protected final GenomeSegment segment; protected final TObjectFloatHashMap spawnList = new TObjectFloatHashMap(); protected Gene(Genome genome, GenomeSegment segment){ this.genome = genome; this.segment = segment; this.genome.addGene(this.segment, this); } void mutate(Random random){ changeSettings(random); spawnNewGenes(random); } /** * 'mutates' the gene * @param random */ protected abstract void changeSettings(Random random); /** * generates an identical copy of this gene and adds it to the specified genome * @param genome * @return */ protected abstract Gene clone(Genome genome); protected final void spawnNewGenes(Random random){ for(GeneFactory factory : this.spawnList.keySet()){ if(this.spawnList.get(factory) >= random.nextFloat()){ factory.createNew(this.genome); } } } /** * Used in the fitness function to favor more interesting constellations. * Returned values should be between 0 and 1. * @return */ protected abstract float getInterestingness(); } |
Let’s look at this in some more detail. The most relevant method from an evolutionary process type of view is the one called ‘mutate’ which calls the abstract method ‘changeSettings’ and the already implemented method ‘spawnNewGenes’. The changeSettings method stays true to the basic idea of evolutionary processes by changing what the gene does ever so slightly. This might result in a small change in properties such as size, speed, color and the like. What these properties all have in common is that they can be expressed as numerical values. This does not however work for behavioral logic blocks such as those controlling movement, aiming and the like. To achieve variability there we use the second method. The ‘spawnNewGenes’ is rather atypical for an evolutionary optimizer as it does not change a gene but adds new ones to the genome. To better understand how this process works it is important to know that there are two fundamentally different types of genes in this simulation, dominant genes and combinable genes. The dominant genes are used on segments where it not clear what a combination of multiple genes would look like. These control, or rather select the logic blocks responsible for the previously discussed behaviors. Dominant genes have each have a method with which dominance in a certain segment can be determined. Once a dominant gene is found in a segment, all other genes are muted. They are still there but don’t have an active effect. We will come back to this later to see why it is relevant. In contrast to the dominant genes, we have the combinable genes. All the combinable genes in a segment work together to determine the final properties of this segment. There might for example be gene which sets the size to a value of let’s say 10, another one which has a +1 effect on size and one which has a +10% effect which would for this example result in a size of roughly 12. But back to ‘spawnNewGenes’. In every generation (which in our case is one per level), a gene has a chance of adding additional genes to the genome. This is important to transition from one dominant gene to another but can also be used to generate more drastic changes in areas controlled by combinable genes. But there is not only the addition of new genes. To simulate that a random mutation might destroy a gene, there is a small chance that with every transition from one generation to the next, a gene might be dropped from the genome. The effect of this is most noticeable when the dropped gene has been the dominant one for its segment. This is also why all the other genes of a segment are kept even if they have been muted. There might be a situation where they become activated again. Extra checks are in place to assure that every segment has at least one active gene in order for the species to be functional. This is another thing which does not happen in biology. But since we want the game to remain being playable we have no other option than to constrain the simulation in certain ways. An additional limitation we imposed comes in the form of what we called ‘interestingness’. There are certain perfectly correct combinations of genes which result in a utterly boring and borderline game-breaking species. While the combination of a long-ranged attack with a ‘no-movement’ behavior might be interesting and a melee attack paired with a swift and sneaky movement might be interesting as well, the combination of melee and sitting still is certainly not. It is the interesingness’s job to if not entirely prevent such situations (there is a lot of random going on after all) at least greatly reduce the probability of their occurrence.
There is one more important aspect of this process which we have not yet discussed so far and this is the so called fitness function. Since evolution is all about the survival of the fittest, meaning the individual (or in our case species) which is best adapted to its environment has the highest chances of survival. This means we need a way to evaluate/estimate the fitness of a species relative to another one. The easiest way to do this would be just to see what is left after the player leaves a level and go from there. There is one major disadvantage with this approach though. Imagine there are two species in a level. One of them just happens to be between the spawn-point and the level exit while the other one is in a part of the level which the player does not necessarily need to visit. Even though the first could be the stronger one or the more interesting one, there will necessarily be fewer of them around once the player goes through. This is why we use a different metric: player interaction. Species score higher the more they interact with the player. They get rewarded for inflicting damage on the player and are penalized (though to a much smaller degree) for taking damage. This has the effect that species which interact with the player a lot thrive while those which do not wither away. To limit the possibilities for the player to drive a species to extinction by simply ignoring it (though this is a valid strategy), individuals are randomly distributed through out the level which makes it more difficult to avoid them deliberately. Doors, delayed spawns and similar mechanics further help to make species avoidance more difficult.
While using such evolutionary processes for entity generation offers a wide range of possibilities, it is important to keep one thing in mind: nature is messy and unpredictable and therefore must any process which tries to simulate it be messy and unpredictable as well, at least to a certain degree. There are countless ways to tune parameters and introduce constrains to guide the process into a rough direction, some of which we discussed here but there are no guaranteed outcomes. This is however kind of the point of this whole exercise since if you wanted per-determined outcomes you could just go ahead and pre-determine them. Getting this process right (which we do by no means claim we have) or at least working (which we want to believe we have) is incredibly time consuming and tedious. The results of such a process might surprise you as they are more often than not different from the initial expectations.

The transition graph showing which weapon genes can spawn which.
Items and Power-ups
As with genes, (and also for the exact same reason) we have two different types of power-ups; the ones modifying (only) stats and the ones modifying abilities (and also stats, because, why not). The first type is dropped by all enemies when they are destroyed and is dependent on their genetic composition. Stronger enemies will drop items which will (slightly) increase the players strength while the drops of especially quick enemies will increase the players speed and so on. These drops are designed in a way that they all have positive influences on several metrics of the player but are most prominent in one. Enemies might, depending on the genes in the ‘drop’ segment of their genome also drop additional power-ups with constant effects such as a +1 health with a certain probability. The player might then try to keep this species alive for as many levels as possible since they can be ‘farmed’ to a certain degree which could have significant positive impacts on the players chances of beating the game.
The other type of power-up is the one which has an effect on the players abilities. They are not spawned by enemies but rather are scattered throughout the game, in most cases there is one per level. To preserve symmetry, these abilities (with very few exceptions) are designed in a way that they work for both the player and the enemies. This has the consequence that every special ability the player encounters when being attacked by an enemy will be available to the player via a power-up eventually. Placement of these power-ups is entirely random with the only constraint that no power-up may be spawned more than once per run.
The inherent randomness of this approach necessitates that the effect of these power-ups, especially the ones with additional abilities, cannot be large in order to avoid the situation where a certain constellation of abilities results in a disproportionally strong species of enemies early in the game. While such combinations exist, we designed the ‘mutation-rate’ in enemies vs the spawn-rate of power-ups available to the player in such a way that it is way more (but still not very) likely for the player to have such a combination than it is for an enemy to ‘evolve’ one.
Visual and Sound effects
A big part of the ‘lore’ of the game, if you want to go so far as to call it that, is conveyed through visual effects which are for the most part realized using a chain of post-processing shaders. These shaders are very simple units which perform either local or global transformations on the rendered image before it is displayed. Implementing the pipeline in this way gives us quite some flexibility for varying the parameters of these transformations (each shader has at least one parameter) which can result in a wide range of different effects. A piece of logic called the ‘ShaderProgression’ takes care of the management of these parameters and changes them according to the player’s progress within the game as well as a fair bit of random supplied by the same random number generator which also influenced the level and the enemy development. A downside of having many configurable modules instead of just one is that, similar to the power-ups, each individual effect has to be somewhat small as not to overpower the whole chain rendering (quite literally in this case) the game unplayable. This also puts an upper-bound on the fanciness which cannot be hand-crafted to an artists visions but have to remain small and compatible. Another disadvantage of this modular chaining is that it’s execution requires quite a bit of pixel fill rate. While this is no problem for modern desktop machines (even ones designed for office work and the like) it turned out to be too taxing for smaller/mobile devices which lead us to drop the support for Android/OUYA which was originally one of the reasons why we built our custom engine.
For the sound design, the original idea was to have a soundtrack comprised of a few base themes each of which would have several extending instrumental tracks which could be layered on top depending on the state of the game to create a dynamic sound experience which would be (somewhat) unique on every play-through. This idea had to be abandoned due to several factors, including not only time- but also technical constraints. The audio components of our engine are not able to provide the accuracy of synchronization and the filter chain necessary to make this work and implementing this kind of logic would have been extremely time consuming and not worth the effort at this point. I still hope to be able to do something like this one day but with an engine which does not have to be built from scratch first. What we now have in terms of audio is quite different. Instead of a soundtrack we opted to have only a sound-scape comprised of between one and three layers of atmospheric background tracks which are selected in every level based on the state of the game.
Things to come
Now that all components of the game are in place, the only thing left to do (aside from some polishing and bug-fixing) is balancing. As already described in here, with this type of game it is pretty much impossible to ensure that every run will be a well balanced experience, at least not without ruining the ‘something slightly different every time’ aspect of the procedural generated world. This goes somewhat against the concept of ‘flow’ which would require a precisely-ish controlled difficulty curve. Something we can do however is tweak the difficulty curve of the average run. To do this, we record certain key indicators throughout game such as speed, health, damage, etc. for both player and enemies and aggregate them over many runs. If the divergence of the values between player and enemies becomes too large at a certain point, changes to the mutation logic which governs enemy development can be made in order to (try to) mediate the problem. This again is a quite time consuming process about which we will certainly have to say some things in the far future, so stay tuned…
a nifty diary #4: Gameplay Preview
As we told you in our previous post, we recently had the first public presentation of the current state of our nifty game. Sadly, there is no video of the festival presentation itself, so we decided to just record another playthrough and put it online for you to see. So here you go, an early look on how the game plays:
We’re doing science and we’re still alive…
…still alive, still alive…
Despite the lack of updates, the project is still being worked on. Over the last couple of months, we changed some things and added some more but the progress was sadly not as big as we hoped for. This is due to the fact that all members of our core development team graduated from university and had to work on their final project. (And yes, we are aware that we haven’t actually introduced ourselves, another item on the ever-growing todo-list.)
We currently can’t really say if there will be more or less time for the development of this game in the future, compared to the last half year or so. As always, we will keep you updated about the progress (eventually). We also hope to have more game-related content to share soon, so stay tuned…
A nifty diary #3: generated enemies in their natural habitat and more
Lots of things happened over the last few weeks, none of which are particularly presentable. Since the blog has been quiet for a while, we’re going to present them anyway.
Feature creep
Feature creep is the scourge of humanity, or at least of software development. We have noticed some of the symptoms of feature creep – mainly the steady increase of features and the lack of increase on things like content – and decided to take action. So we defined a set of missing features which are absolutely vital for the game and we are determined to implement only those at least until we have a demo to show (wish us luck). We will post about those features as soon as there is something to show.
Data structures, memory and concurrency
The grid, the core data structure which handles everything contained within a level, has been updated yet again. The current (and hopefully final) version uses a sparse implementation and pointers to the position of an object within the grid. This allows for a delayed and completely concurrent access to every object. With this change we were able to separate steps of the update of objects which had to be executed consecutively before. This finally led to the possibility of a parallel game loop.
Parallel updates
Most CPUs nowadays have more than one core. This is great when you want to do multiple things at once but makes it difficult do utilize the full power of a CPU in one application. This is why even today, a lot of games are not parallelized to the degree necessary to do so. With the latest changes, we made a step into that direction. The logic of the game loop which calculates the behaviour of every game object in every frame is now capable of using multiple cores at once which should lead to an improvement of the framerate on lower end CPUs. Due to the architecture of OpenGL, it won’t be possible to parallelize the draw logic as well, so we won’t be able to use all the computing power which is theoretically available.
Procedural enemies
The enemy generator described in this post is now integrated into the game. While it does currently not unfold its true potential, it is still interesting to watch. We also added a similar but simpler algorithm for generating swarm enemies. The current state of this endeavour is demonstrated in the video below.
Procedurally generated enemies
As you may or may not know, one of NIFTY’s core mechanics (if you want to call it a mechanic) is random/procedural generation of stuff. We’ve been discussing quite a bit about what parts of the game should be generated and what should be just fixed (balance between time consumption and awesomeness). One of many aspect we really liked but didn’t know if it was feasible was generation of enemies because of the huge amount of expected time we would need to implement it. As it turns out, generating sprites (and models from these sprites) is way simpler than we dared to dream. It only took 1 hour from an idea to a working prototype and a few more hours to get the whole thing to a useable stage.
Idea
First some restrictions for our generated sprites were necessary to make the generation easier and to get a rough picture of what we want. The most important restriction comes from the idea that these sprites should look like they represent something and what does the human brain like to find the most in random things? Faces. Simply put, everything that has a symmetry and some kinds of dots in it looks like a face to us. Be it animal, monster or robot, if it has a face we know we can interact with it. That said, our first restrictions is that the sprites needs to be symmetrical. Our second restrictions is as simple as our first one, we want all pixels in the sprite to be connected to each other.
The second restriction can easily be satisfied by using an algorithm which builds on already set pixels and ‘grows’ them into a sprite.
Algorithm
Enough text for now, let’s start with some more specific instructions:
- Set a seed point in the middle of the sprite
- While not enough pixels set do:
- Randomly select a pixel that’s next to a set pixel
- Compute probability for setting this pixel (decision function)
- Set the pixel using the probability from 2.B
- Profit!
Well that’s it. Nothing spectacular here. One bit of magic is hidden in the decision function
but even if it always returns a constant value it already generates nice images:
By adding some lighter and darker spots (again at random) and changing the decision function we can influence the generated sprites more. In our case we want some ability scores (health, speed, power) to influence the look of the sprites so the player can estimate what type of enemy he’s looking at. Here are some examples of what the generator spits out in it’s current form:
High speed: small, high detail
High power: medium size, high detail, more light/dark spots
Code excerpt
Here are two code snippets (in Java) from our implementation to give you some idea how it is implemented in NIFTY.
Note: the openList for storing points that haven’t been checked yet and the closedList for points that have been check are both of type HashSet<Position> .
Generation loop:
1 2 3 4 5 6 7 8 9 10 11 12 13 14 15 16 17 | public void generate() { long seed = r.nextLong(); r = new Random(seed); long time = System.currentTimeMillis(); clear(); int iterations = 0; for (int count = 0; count < (w * h / 2) * fillPercentage; ++iterations) { count += grow() ? 1 : 0; // count amount of pixels set } addDecoration(); System.out.println("Runtime: " + (System.currentTimeMillis() - time) + "ms"); System.out.println("Iterations: " + iterations); System.out.println("Random seed: " + seed); } } |
Growing step:
1 2 3 4 5 6 7 8 9 10 11 12 13 14 15 16 17 18 19 20 21 22 23 24 25 26 27 28 29 30 31 32 33 34 35 36 37 38 39 40 41 42 43 44 45 46 47 48 49 50 51 52 53 54 55 56 57 | public boolean grow() { // System.out.println("Open list size: " + openList.size()); // System.out.println("Closed list size: " + closedList.size()); int xx, yy; Position p; Iterator it; do { it = openList.iterator(); p = it.next(); // Randomly pick element int num = r.nextInt(openList.size()); for (int i = 0; i < num; ++i) { p = it.next(); } xx = p.getX(); yy = p.getY(); if (pixels[map(xx, yy)] != PColor.EMPTY) { // Pixel is already set // Move to closed list it.remove(); closedList.add(p); } } while (pixels[map(xx, yy)] != PColor.EMPTY); int countSide = 0, countDiag = 0; for (int ix = xx - 1; ix <= xx + 1; ++ix) { for (int iy = yy - 1; iy <= yy + 1; ++iy) { if (ix < 0 || ix >= w || iy < 0 || iy >= h / 2) // outside drawing range continue; if (pixels[map(ix, iy)] != PColor.EMPTY) { if (ix == xx ^ iy == yy) ++countSide; else ++countDiag; } } } // Check if we should set this pixel if (r.nextFloat() < evaluator.getPixelSetChance(countSide, countDiag)) { pixels[map(xx, yy)] = PColor.NORMAL; // Move point to closed list it.remove(); closedList.add(p); // Add surrounding points to open list addPoint(xx + 1, yy); addPoint(xx - 1, yy); addPoint(xx, yy + 1); addPoint(xx, yy - 1); return true; } return false; } |
This enemy generator is neither finished nor integrated in the game yet, and the colors need some tweaking as well. We hope to be able to show the first completely procedurally generated enemies within the game soon…
A nifty engine
Every video game these days is powered by a game engine. It provides the basic functionality needed to make the game work. And while there are some engines out there which we could have used, we decided to build our own: the TopHatEngine.
We build T.H.E for several reasons. We needed access to various levels of the engine from within the game to be able to achieve some of the effects you are going to see some time in the future. It also allows us to optimize some of the inner workings to our voxel-type artstyle, which is neither really 2D nor 3D in the traditional sense. Lastly, we want the game to run on PC, Android and OUYA. These different platforms bring various limitations and even though there are some commercially available engines out there which run on all of these platforms, we were not convinced that we could do everything we wanted in the game using one of these. So T.H.E is composed of various modules which can be combined in such a way to accommodate for the different peculiarities of the respective platforms. Like the game itself, T.H.E is still a work in progress, so expect some updates about it in the future…
A nifty diary #2: weapon system
It’s been a while… one of us, the developer who was working on the weapon system, had to step back from the project due to time management conflicts. This caused some changes in the overall schedule which caused the lack of posts lately.
But now, without further ado, we present our nifty little weapon system. There are four different weapon types (well, five actually if you count melee): shot, grenade, rocket and laser. The properties of all these weapons can be modified through various pickups and the like. Here’s a short overview:
OUHYAAAAH!
After many hours of debugging of our OUYA port I found the very sneaky bug that’s been bugging (ha!) me for the whole time. As it turns out the Tegra 3 (which is the core used by OUYA) can only handle textures of sizes that are a power of 2 (there are still GPUs that can’t handle arbitrarily sized textures… go figure). Anyhow, Nifty is now running on the OUYA (the startscreen that is) although the scaling up to 1080p isn’t yet working as expected…